Statistics
Here you can find lists of great Statistics books recommended by some of top experts form best universities and leading technology companies.
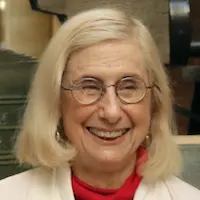
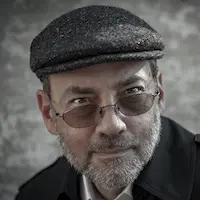
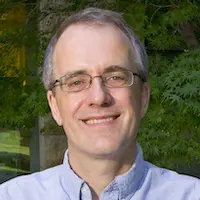
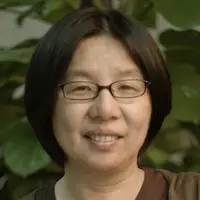
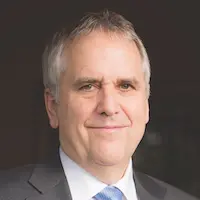
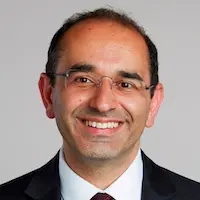
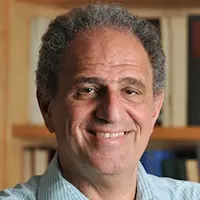
Statistics is an important mathematical field with applications in engineering and science, as it provides a set of tools and techniques that can be used to analyze data and make decisions. At its core, statistics is the process of collecting, analyzing, and interpreting data in order to draw conclusions and make predictions. In this article, we will explore the basics of statistics, including the different types of data, the different types of statistical techniques, and the applications of statistics in various fields of electrical engineering.
There are two main types of data that are typically analyzed in statistics: continuous data and categorical data. Continuous data is data that can take on any value within a certain range, such as temperature or weight. Categorical data is data that can only take on a limited number of values, such as color or gender.
One of the most important statistical techniques used in electrical engineering is descriptive statistics. This is the process of summarizing and describing data in a concise and meaningful way. Descriptive statistics includes measures of central tendency, such as mean, median, and mode, as well as measures of spread, such as variance and standard deviation.
Another important statistical technique is inferential statistics. This is the process of making predictions or drawing conclusions about a population based on a sample of data. Inferential statistics includes techniques such as estimation, hypothesis testing, and regression analysis.
Statistics is also used in the field of signal processing. Signal processing is the process of manipulating and analyzing signals in order to extract useful information. Statistics plays an important role in signal processing by providing techniques for characterizing and modeling signals, such as probability density functions and autoregressive models.
In the field of control systems, statistics plays an important role in the design and analysis of control systems. Statistical techniques such as linear regression and Bayesian estimation are used to model and control systems. Also, statistical process control (SPC) is a method of using statistical techniques to monitor and control a process, it’s a powerful tool to detect and correct process variability.
In the field of communication systems, statistics is used to improve the performance and reliability of communication systems. Techniques such as error correction and data compression are used to improve the quality of communications systems, while modulation and demodulation are used to transmit signals over different types of channels.
In the field of machine learning, statistics plays a critical role in the development of algorithms and models that can be used to analyze and make predictions from data. Techniques such as supervised learning, unsupervised learning, and deep learning are used to develop models that can be used to analyze and make predictions from data.
In conclusion, statistics is an important field in electrical engineering, as it provides a set of tools and techniques that can be used to analyze data and make decisions. The different types of data, the different types of statistical techniques, and the applications of statistics in various fields of electrical engineering are all important considerations when working with data. Statistics is critical for signal processing, control systems, communication systems, machine learning, and many other fields.